Part 2 – Tracking Motion
While I was thinking about which way to research this approach, it set me thinking about what motion was, or more specifically, what is a computer’s idea of motion.
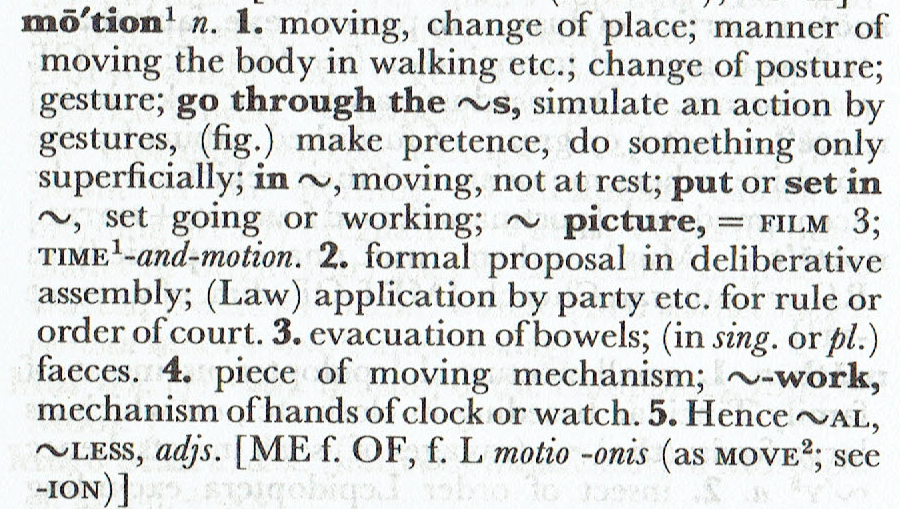
Definition of Motion taken from OED
The answer would be that it would not understand motion, it would detect that the information contained within an image may have changed in comparison to the next one in a sequence but all we are talking about are specific 2D or 3D values associated with individual pixels within a specific colour space.
So to achieve a result that we would recognise as motion the computer has to take the pixel values and analyse the change in values in a way that we can use for tracking movement.
Centroid (find the centre of a blob)
So what is a blob exactly? in image processing terminology a blob is apparently a group of connected pixels sharing common values, and the centroid in this instance is the mean or average of this blob or the weighted average if you will.
OpenCV finds this weighted average with what is known as ‘moments’ by converting the image to grayscale, then perform binarisation before conducting the centroid calculations.
Multiple blobs can can be detected using this method within OpenCV with the aid of ‘contours’.
Meanshift & Camshift
Meanshift and Camshift can be used in conjunction with Centroid for the purposes of locating the part of the image we need to track.
Meanshift is a non-parametric density gradient estimation. It is useful for detecting the modes of this density. Camshift combines Meanshift with an adaptive region sizing step.
Both Meanshift and Camshift use a method called histogram back projection which uses colour as hue from a HSV model.
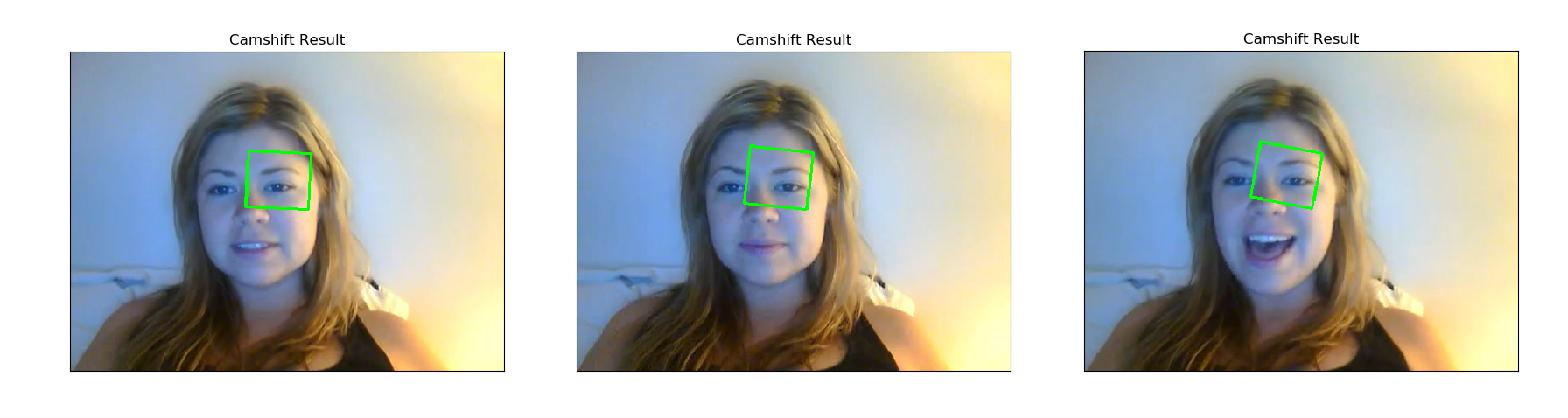
Results of my Camshift experiment on our allocated images.
Code for my Camshift program:
1 2 3 4 5 6 7 8 9 10 11 12 13 14 15 16 17 18 19 20 21 22 23 24 25 26 27 28 29 30 31 32 33 34 35 36 37 38 39 40 41 42 43 44 45 46 47 48 49 50 51 52 53 54 55 56 57 58 59 60 61 62 63 64 65 66 67 68 69 70 |
import sys import numpy as np import cv2 from matplotlib import pyplot as plt import easygui # Code written by Gavin Morrison D12124782 # Opening an image using a File Open dialog: # f = easygui.fileopenbox() # I = cv2.imread(f) # Import image to be treated original_image = cv2.imread("Ilovecat1.bmp") # Copy of image to isolate region of interest tracking_image = cv2.imread("Ilovecats1Copy.bmp") # Coordinates of region of interest [row:row, column:column] region_of_interest = tracking_image[125: 160, 210: 250] # Coordinates for right eye # region_of_interest = tracking_image[125: 175, 145: 200] # The region of interest is converted to HSV colour space HSV_region_of_interest = cv2.cvtColor(region_of_interest, cv2.COLOR_BGR2HSV) #Create histogram of region of interest, using hue. The hue range is from 0 to 179. region_of_interest_histogram = cv2.calcHist([HSV_region_of_interest], [0], None, [180], [0, 180]) #Original image is converted to HSV colour space original_image_HSV = cv2.cvtColor(original_image, cv2.COLOR_BGR2HSV) # Back projection used to create mask from the hue of the region of interest histogram mask = cv2.calcBackProject([original_image_HSV], [0], region_of_interest_histogram, [0, 180], 1) # Filter applied in an attempt to reduce noise in the mask (later addition) filter_kernel = cv2.getStructuringElement(cv2.MORPH_ELLIPSE, (3, 3)) mask = cv2.filter2D(mask, -1, filter_kernel) _, mask = cv2.threshold(mask, 10, 255, cv2.THRESH_BINARY) # The coordinates of our region of interest are assigned to variables row = 210 column = 125 width = 250 - row height = 160 - column # Coordinates for right eye # row = 145 # column = 125 # width = 200 - row # height = 175 - column # define criteria criteria = (cv2.TERM_CRITERIA_EPS | cv2.TERM_CRITERIA_COUNT, 10,1) # rectangle of tracking area is created using camshift function rectangle, tracking_area = cv2.CamShift(mask, (row, column, width, height), criteria) #These are the points for the rectangle points = cv2.boxPoints(rectangle) points = np.int0(points) # cv2.polylines used instead of 'cv2.rectangle' to accommodate rotation of bound space. cv2.polylines(original_image, [points], True, (0, 255, 0), 2) # Display our processed image finished_image = cv2.cvtColor(original_image, cv2.COLOR_BGR2RGB) plt.subplot(111),plt.imshow(finished_image,cmap = 'gray'), plt.title('Camshift Result'), plt.xticks([]), plt.yticks([]) plt.show() |
REFERENCES:
Find the Center of a Blob (Centroid) using OpenCV (C++/Python) (accessed 2nd November 2018)
https://www.learnopencv.com
Meanshift and Camshift (accessed 02.11.2018)
https://docs.opencv.org/3.4.3/db/df8/tutorial_py_meanshift.html
Mean Shift Tracking (accessed 02.11.2018)
https://www.bogotobogo.com/python/OpenCV_Python/python_opencv3_mean_shift_tracking_segmentation.php
Back Projection (accessed 02.11.2018)
https://docs.opencv.org/2.4/doc/tutorials/imgproc/histograms/back_projection/back_projection.html
Bradski GR. Computer Vision Face Tracking For Use in a Perceptual User Interface. Microcomputer Research Lab, Santa Clara, CA, Intel Corporation.
http://citeseerx.ist.psu.edu/viewdoc/download;jsessionid=B9A5277FF173D0455494A756940F7E6B?doi=10.1.1.14.7673&rep=rep1&type=pdf